How Many Piano Tuners in Lincolnshire? How Evolved AI® helps us answer seemingly impossible questions.
Tuning Into Problem Solving: The Fermi Challenge from Chicago to Lincolnshire?
Evolved AI® Answers Impossible Questions
So, how many piano tuners are there in Lincolnshire? Take a few seconds to make a guess. Pretty difficult, no? If you’re trying to ‘work out how to work it out’, you’re in good company. People who’ve tackled this question before range from new data scientists to students from the University of Lincoln… to Nobel Prize winners [1].
Italian-born American scientist and Nobel Prize winner Enrico Fermi is hailed as one of the most important nuclear scientists of all time [2]. Now a little more famous as a character in the celebrated movie Oppenheimer, Fermi began his career as a mathematician and statistician and developed his famous problem ‘How many Piano Tuners are there in Chicago?’ whilst teaching at the University of Chicago [1,2]. The premise was to help students learn to work towards an unknown solution [3]. The students are asked to think about what things they need to know to answer the question [4]. Then, using information that can be easily obtained (such as the population of the city) and logical reasoning, they are able to make assumptions about the answers that are not so easy to obtain [4]. The approach focuses on combining those estimates to create an answer to an otherwise ‘unknown’.
Doug Hubbard, an American decision scientist, expanded on Enrico Fermi’s method of estimation with his development of Applied Information Economics (AIE) [5]. This approach integrates Fermi’s techniques with advanced statistical methods, such as Monte Carlo simulations and Bayesian analysis, to address complex problems in various fields, including finance and policy analysis [6,7].
This problem is now used as an interview question by universities, consultancies, and tech companies alike to understand a candidate’s problem-solving and critical-thinking skills [8]. At Lone Star Analysis (LSA), this problem is used to train all our new starters.
Back in September, Lone Star Analysis had a slot/stall/starring role at the University of Lincoln careers fair. We wanted to do something fun. So rather than asking students to ‘Guess the Number of Sweets in a Jar?’ we instead asked students, ‘How many Piano Tuners are there in Lincolnshire?’.
Evolved AI® to the Rescue
Lone Star Analysis (LSA) is an international company that specialises in applied decision intelligence. We provide predictions and develop solutions injected with Evolved AI® to help people make smarter decisions. Often these decisions involve problems which are highly complex, surrounded by uncertainty, have missing or poor data, and the outcomes are mission critical. LSA is successful at this due to its ability to account for uncertainty. It’s helped our customers tackle big challenges across a spectrum of problems, including in defence, healthcare, and sustainability – we’ve even worked for NASA [9].
LSA starts with software that formalises Fermi’s principle – asking for the Big Question, which can be answered by a series of Little Questions®. By working backward (with the people who need to know the answer), it is possible to quickly understand the key factors that influence the final answer – and their interrelationships within the problem.
But going beyond even Fermi, at LSA, we recognise that both the present and the future isn’t one fixed point but is a range of possibilities. Thus, when accounting for each influencing factor, LSA ask five key questions:
- What would the answer be typically?
- What would it be as low as 1 in 10 times?
- What might it be as high as 1 in 10 times?
- What will it never be lower than?
- What will it never be higher than?
This gives us what is known as a ‘ten base ninety’ (10B90). Our TruNavigator MAX™ platform runs hundreds of thousands of simulations, randomly selecting from every single possible value within the range of uncertainty for every single influencing factor. If a factor is unknown, we make the best possible estimate – using the feasible assumptions – this allows us to accept uncertainty. This gives us a prediction that is more likely to reflect future reality, as well as giving us a prediction that itself has a range. By simulating all the possible combinations of this uncertainty, we can understand the most important influencing factor in making the decision. So, for the piano tuner example, you could work out what factor is driving that final prediction – and what’s most uncertain at the same time.
Taking this approach further, you can even set goals (and constraints) and then get the machine to ‘work backward’ to tell you what 10B90 each influencing factor would need to be to achieve that goal. This is our ‘Evolved AI®’ (or, to use the more technical term, Adaptive Non-convex Stochastic Optimisation), and this approach is at the heart of how all LSA solutions and software operate.
So how many Piano Tuners *are there really* in Lincolnshire?
It was this practice that inspired LSA UK to set a unique challenge for students at The University of Lincoln Careers Fair. After a brief tutorial on how the LSA method works, we asked students to think through the influencing factors and make some guesses about them – and then make an educated guess based on that. To help them think things through, we then showed them the model in Figure 1. We received a range of answers from dozens of students guessing that there was only one solitary piano tuner in Lincolnshire to over a million!
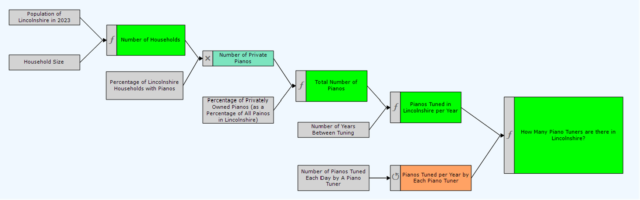
Figure 1: Piano Tuners in Lincolnshire
Once the day was over, it was then up to us as the ‘experts’ to decide on the competition winner. But this left us with a problem – the point of this exercise, when LSA is training (and for Fermi’s students 100 years ago), was that there ‘is no right answer’ – it was just about learning to break things down by determining degrees of confidence and ranges in which the answer is likely to fall. But this time, it was up to us to find the ‘right’ answer.
We started by looking for the data that we knew existed, beginning with 2021 Census data, which we extrapolated to give an estimate of the current population of Lincolnshire [10]. We went with how the Census defined Lincolnshire; Boston, East Lindsey, Lincoln, North Kesteven, South Holland, South Kesteven, and West Lindsey. Household size in the census was also derived from this location [10]. Unfortunately, this was the only ‘hard’ data that we were able to get from online research, leaving the remainder of the data to be collected from Subject Matter Experts (SMEs).
We did a search for the piano tuning shops/services based in Lincolnshire (as provided by Google, Yelp, and other online searches) and got an initial single number – but knew that we could go one step further. So, after some phone calls with some confused but very helpful piano tuners, we were able to collect estimates for most of the remaining data points. Again, we used a 10B90. With the assistance of our TruNavigator MAX™ model in Figure 1, we managed to create a range, including a possible and likely, for the number of Piano Tuners in Lincolnshire – Figure 2.
Results:
The use of the two different methods – speaking to experts and using the TruNavigator Max™ model allowed us to create a range for the number of piano tuners in Lincolnshire – Figure 2.

Figure 2: Piano Tuners in Lincolnshire Estimate
Figure 2 shows the possible and likely range of the number of piano tuners in Lincolnshire as well as the most likely answer. Using TruNavigator MAX™, we can also present this data in a graph – Figure 3.
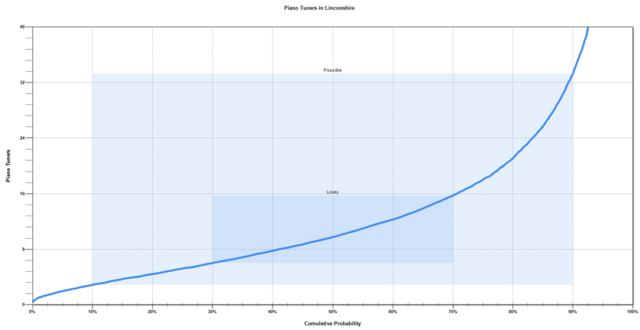
Figure 3: Graph to Show Piano Tuners in Lincolnshire
From Figure 3, the likely and possible range is shown clearly; this enabled us to select a winner for our competition, as seen in Figure 4.

Figure 4: Winning Participant – Who Guessed the Number of Piano Tuners in Chicago Most Accurately?
The Fine-Tuning
Despite the success of this challenge, there were a number of distinct issues with this problem and how it is solved that are an inherent part of Fermi’s problems. These are due to the demographics and geography of the original problem. In addition to these issues, the way in which the facilitator interprets and presents the problem will also impact the way in which the question is answered.
Firstly, as discussed previously, the point of these questions is not to elicit a correct answer but to go through the process of estimating – ‘making reasonable assumptions under conditions of uncertainty’. Although, as the ‘experts’ we used our best knowledge to really fine-tune the answer, essentially, this challenge is not something that we can really ‘know’ the answer to.
The geography of any question is always important, and here its relevance centres around the differences between these two locations. Chicago is a large US city with a well-defined boundary. In contrast, Lincolnshire is in the UK and can be defined as both a region and a county.
Upon discussion with multiple piano tuners who are based in Lincolnshire, it became apparent that not all Pianos are tuned frequently, with some having seen pianos that haven’t been tuned in over ten years. In contrast, Chicago is known as a global music leader – and hence, may tune pianos more frequently. In addition to this factor, it is important to note the possible high values in Figures 2 and 4. The modelled value is much higher than the value stated by the winner of the competition. This is due to an overestimation in the number of piano tuners in Lincolnshire caused by the diversification of piano tuners, who, in most cases, perform multiple duties outside of tuning pianos.
The impact of bias is a concern for any research project, in particular when it involves the answering of questions. Here the way in which the facilitator understands, asks, and records the questions can have a large impact on the results of the investigation. These three questions provide a good example of bias:
- What speed was the car travelling before it came to a stop?
- How fast was the car going before it came to a stop?
- How fast was the car travelling before it screeched to a halt?
Although the right answer to each of these questions is the same, this ‘framing effect’ can influence the participant into answering each question differently.
Research also shows that the mathematics training of the individual can also have a significant impact on the understanding and answers of the participants. Studies even go as far as to say that the more mathematical training you have, the less likely you are to be able to cope with basic probabilities.
Asking someone, ‘What percentage of houses have a piano?’ might elicit a different response from ‘Out of 100 randomly selected houses, how many have a piano?’ Similarly, ‘What is the 90th percentile in years between each tuning for all the pianos in Lincolnshire’ might again be very different from the more relaxed ‘If you went from piano to piano looking at years between tuning, for one in ten pianos, what might a high number look like?’.
In line with the fact that there is no ‘right’ answer to this question as a learning exercise is the issue of how a ‘winner’ was chosen. We could have picked the candidate with the most accurate upper bound, most accurate lower bound, or the most accurate median. Each of these methods would have given us a different winner. Instead, we decided to pick the candidate with the most accurate likely and possible ranges – simply because that is the LSA way!
Harmonising the Unknowable
So how do you answer a non-answerable question? You can only try, but regardless of the industry, the complexity – or even the uncertainty of the question, Lone Star can help.
References
- https://www.nobelprize.org/prizes/physics/1938/fermi/biographical/
- https://www.britannica.com/biography/Enrico-Fermi
- https://engines.egr.uh.edu/episode/2170
- https://physics.wm.edu/~sher/fermi
- https://hubbardresearch.com/publications/how-to-measure-anything-book/
- https://www.investopedia.com/terms/m/montecarlosimulation.asp
- https://www.nature.com/articles/s43586-020-00001-2#:~:text=Bayesian%20statistics%20is%20an%20approach%20to%20data%20analysis%20and%20parameter,the%20prior%20and%20data%20distributions.
- https://ram921.medium.com/answering-unanswerable-questions-fermi-problems-in-product-management-interviews-30d2806da094
- https://lone-star.uk/
- https://loric.bgu.ac.uk/knowledge/greater-lincolnshire-and-rutland-2021-census-demographics#:~:text=A%20first%20look%20at%20the,across%20Greater%20Lincolnshire%20and%20Rutland